Highlights:
Synthetic Data Generation with Large Language Models for Personalized Community Question Answering
10/29/24
Source:
Braga et al., University of Milano-Bicocca, Milan, Italy
Research
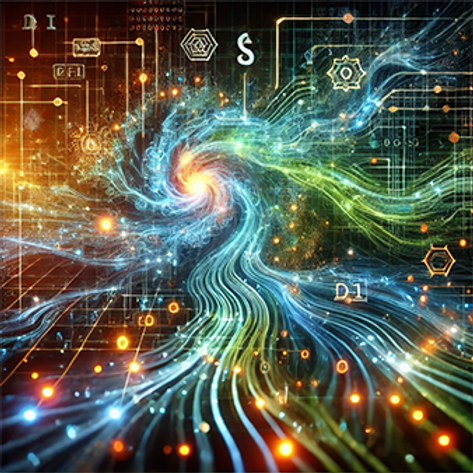
Personalization in Information Retrieval (IR) is a topic studied by the research community since a long time. However, there is still a lack of datasets to conduct large-scale evaluations of personalized IR; this is mainly due to the fact that collecting and curating high-quality user-related information requires significant costs and time investment. Furthermore, the creation of datasets for Personalized IR (PIR) tasks is affected by both privacy concerns and the need for accurate user-related data, which are often not publicly available.
Recently, researchers have started to explore the use of Large Language Models (LLMs) to generate synthetic datasets, which is a possible solution to generate data for low-resource tasks. In this paper, we investigate the potential of Large Language Models (LLMs) for generating synthetic documents to train an IR system for a Personalized Community Question Answering task.
To study the effectiveness of IR models fine-tuned on LLM-generated data, the authors introduce a new dataset, named Sy-SE-PQA. The authors build Sy-SE-PQA based on an existing dataset, SE-PQA, which consists of questions and answers posted on the popular StackExchange communities. Starting from questions in SE-PQA, the authors generate synthetic answers using different prompt techniques and LLMs. The authors findings suggest that LLMs have high potential in generating data tailored to users' needs. The synthetic data can replace human-written training data, even if the generated data may contain incorrect information.
Latest News