Highlights:
Privacy-Preserving Customer Support: A Framework for Secure and Scalable Interactions
12/10/24
Source:
Awasthi, A. et al (https://doi.org/10.48550/arXiv.2412.07687)
Research
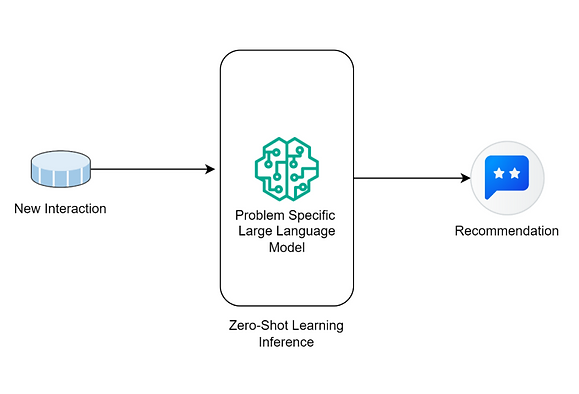
The growing reliance on artificial intelligence (AI) in customer support has significantly improved operational efficiency and user experience. However, traditional machine learning (ML) approaches, which require extensive local training on sensitive datasets, pose substantial privacy risks and compliance challenges with regulations like the General Data Protection Regulation (GDPR) and California Consumer Privacy Act (CCPA). Existing privacy-preserving techniques, such as anonymization, differential privacy, and federated learning, address some concerns but face limitations in utility, scalability, and complexity. This paper introduces the Privacy-Preserving Zero-Shot Learning (PP-ZSL) framework, a novel approach leveraging large language models (LLMs) in a zero-shot learning mode.
Latest News