Highlights:
Exploring Community Detection Methods and Their Diverse Applications in Complex Networks: a comprehensive review
6/12/24
Source:
Khawaja F., et al on Springer Link
Research Review
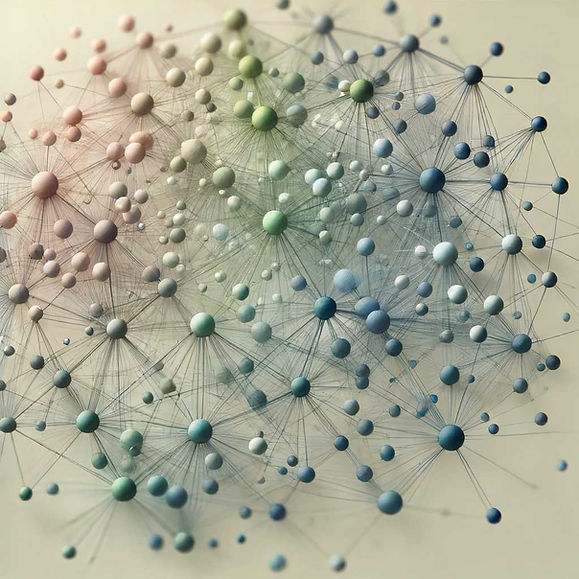
Network science has made tremendous advances, allowing the modeling of complex real-world systems. Although networks include sophisticated community structures by definition, discovering and understanding these communities remains a challenging endeavor, compounded by the need to navigate a multidisciplinary terrain. This comprehensive survey serves as a contemporary guide and systematically presents an up-to-date survey of community detection methods, meticulously categorizing them for a comprehensive understanding. Through critical analysis, we assess the strengths, weaknesses, and performance metrics of various algorithms, ranging from classical techniques to cutting-edge methods designed to address the complexities of overlapping community detection. Additionally, we explore emerging trends in dynamic community detection, including techniques like Temporal Motif Analysis and Continuous-Time Models. We additionally present an in-depth evaluation of these methods, examining their performance on both artificial benchmarks and real-world networks. This review also sheds light on the diverse applications of community detection across domains such as sociology, biology, education, and technology. By presenting a holistic view of community detection, we aim to facilitate researchers’ and practitioners’ access to this crucial field, addressing the theoretical and practical application gaps, and contributing to the continued evolution of network science.
Latest News